
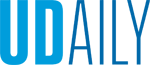

A game-changing tool
Photo by Ashley Barnas Larrimore April 15, 2025
UD’s AI-powered model predicts post-concussion injury risk in college athletes
Athletes who suffer a concussion have a serious risk of reinjury after returning to play, but identifying which athletes are most vulnerable has always been a bit of a mystery, until now.
Using artificial intelligence (AI), University of Delaware researchers have developed a novel machine learning model that predicts an athlete’s risk of lower-extremity musculoskeletal (MKS) injury after concussion with 95% accuracy. A recent study published in Sports Medicine details the development of the AI model, which builds on previously published research showing that the risk of post-concussion injury doubles, regardless of the sport. The most common post-concussive injuries include sprains, strains, or even broken bones or torn ACLs.
“This is due to brain changes we see post-concussion,” said Thomas Buckley, professor of kinesiology and applied physiology at the College of Health Sciences.
These brain changes affect athletes’ balance, cognition, and reaction times and can be difficult to detect in standard clinical testing.
“Even a minuscule difference in balance, reaction time, or cognitive processing of what’s happening around you can make the difference between getting hurt and not,” Buckley said.
How AI is changing injury risk assessment
Recognizing the need for enhanced injury reduction risk tools, Buckley collaborated with colleagues in UD’s College of Engineering, Austin Brockmeier, assistant professor of electrical and computer engineering, and César Claros, a fourth-year doctoral student; Wei Qian, associate professor of statistics in the College of Agriculture and Natural Resources; and former KAAP postdoctoral fellow Melissa Anderson, who’s now an assistant professor at Ohio University. To assess injury risk, Brockmeier and Claros developed a comprehensive AI model that analyzes more than 100 variables, including sports and medical histories, concussion type, and pre- and post-concussion cognitive data.
“Every athlete is unique, especially across various sports,” said Brockmeier. “Tracking an athlete’s performance over time, rather than relying on absolute values, helps identify disturbances, deviations, or deficits that, when compared to their baseline, may signal an increased risk of injury.”
While some sports, such as football, carry higher injury risk, the model revealed that individual factors are just as important as the sport played.
“We tested a version of the model that doesn’t have access to the athlete’s sport, and it still accurately predicted injury risk,” Brockmeier said. “This highlights how unique characteristics—not just the inherent risks of a sport—play a critical role in determining the likelihood of future injury,” said Brockmeier.
The research, which tracked athletes over two years, also found that the risk of MSK injury post-concussion extends well into the athlete’s return to play.
“Common sense would suggest that injuries would occur early in an athlete’s return to play, but that’s simply not true,” said Buckley. “Our research shows that the risk of future injury increases over time as athletes compensate and adapt to small deficits they may not even be aware of.”
From research to real-world injury reduction
The next step for Buckey’s Concussion Research Lab is to further collaborate with UD Athletics’ strength and conditioning staff to design real-time interventions that could reduce injury risk.
Dan Watson, deputy athletic director of competitive excellence and campus recreation, said the AI model can help them target high-risk athletes and incorporate strategies to reduce injury risk.
“In sport performance, we have two goals: improve the athlete's abilities in their sport and to keep them on the field,” said Watson.
UD Athletics already uses force plates to analyze movement and detect muscle imbalances or weaknesses, the leading cause of soft tissue injuries. Watson says that the same proactive approach applies to concussion-related deficits.
“We’re very open to anything that keeps our athletes healthy and on the field,” said Watson. “When this predictive learning model identifies a deficit, we can proactively implement corrective measures.” We can’t prevent injuries, but we can reduce and mitigate the risks, and that’s what this model does for athletics.”
Beyond sports: AI’s potential in aging research
The implications of the UD-developed machine-learning model extend far beyond sports. Brockmeier believes the algorithm could be used to predict fall risk in patients with Parkinson’s disease.
Claros is also exploring how the injury risk reduction model can be applied to aging research with the Delaware Center for Cognitive Aging.
“We want to use brain measurements to investigate whether baseline lifestyle measurements such as weight, BMI, and smoking history are predictive of future mild cognitive impairment or Alzheimer’s disease,” said Claros.
Contact Us
Have a UDaily story idea?
Contact us at ocm@udel.edu
Members of the press
Contact us at 302-831-NEWS or visit the Media Relations website