
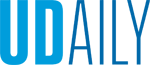
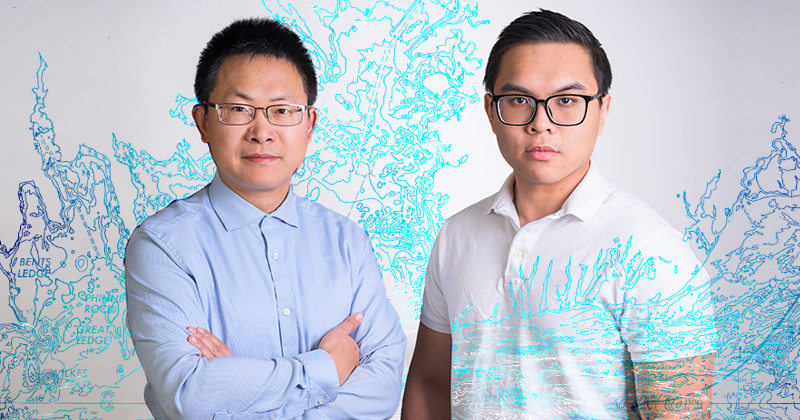
Explainable AI
Photo illustration by Jeffrey C. Chase | Photos by Evan Krape January 29, 2024
UD researchers leverage trustworthy AI to improve seafloor data intelligence
Artificial intelligence and machine learning are tools that have shown great promise in helping humans make deeper connections between disparate data. This is because AI and machine learning models are good at sifting through and analyzing large datasets to detect nuances that humans often cannot see.
Maybe it’s Spotify’s ability to suggest you might enjoy the musical artist Jack Johnson because you often stream the sun-drenched lyrics of Jimmy Buffett. It could be your personal voice assistant’s ability to quickly calculate how many tablespoons are in a quarter cup, with no math required on your part. Those are easy examples to understand.
But these powerful tools are capable of much more complex tasks.
Take the ocean. While the ocean covers 70% of the earth, less than 25% of the ocean’s seafloor has been mapped. This means there is precious little data on this important global resource. Meanwhile, field data collection is expensive and time-consuming, so squeezing as much use out of each available dataset is both important and economical.
Better understanding of the ocean’s seafloor could inform everything from how and where storms develop to how climate change will affect different regions of the world.
Now, with funding from the Department of Defense, University of Delaware computer scientist Xi Peng and oceanographer Art Trembanis are using artificial intelligence and machine learning to analyze seafloor data from the Mid-Atlantic Ocean. The goal is to develop robust machine-learning methods that can accurately and reliably detect objects in seafloor data.
“We’re trying to understand what we call morphodynamics, which is how the seabed changes in relation to the physical processes that are occurring from storm waves and tidal currents,” he said. “We have these big datasets, but it's hard to go through them, so we need tools to help us unlock the dynamics of the system.”
A secondary priority is to help people better understand how AI machine-learning models make decisions. For example, when considering seafloor data, how does the model distinguish if an object is a scallop or piece of coral, rather than an unexploded ordnance? These are topics of interest to both the Navy and shipping industries.
In particular, the researchers hope to illuminate how the model can correctly predict when the data has a lot of variation, over space or time. The three-year project is part of $18 million in funding awarded under the Defense Established Program to Stimulate Competitive Research (DEPSCoR) to strengthen basic research in underutilized states and territories.
Why it matters
According to Peng, a faculty member in the Department of Computer and Information Sciences and UD’s Data Science Institute, as well as project principal investigator, making AI more transparent can help increase the trust of applying machine learning for high-stakes applications.
“We want a model that is reliable for unseen scenarios and that can also provide explanations for its decision-making,” Peng said. “We want to develop a generalizable machine-learning method that can be widely applicable across a variety of projects or industries.”
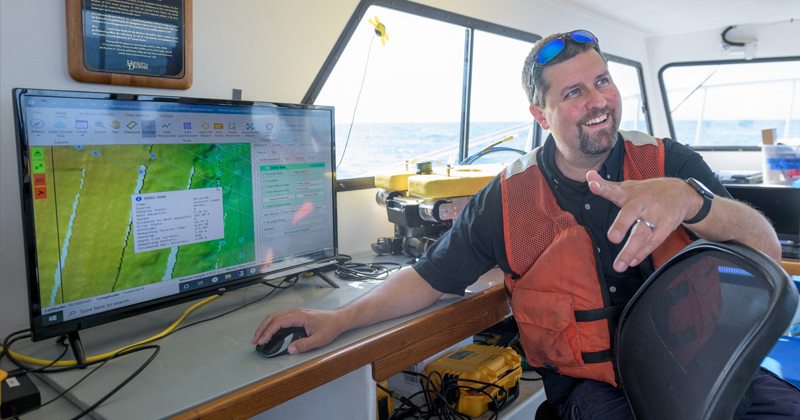
Part of the challenge of mapping complex, diverse systems in the marine environment is that the ocean is a harsh setting and conditions can change quickly — and over time. This means that even in places where the ocean already has been mapped, researchers must consider when it was last mapped.
“When you map something in the ocean, it's like a dairy product. There is a use-by date that is not printed anywhere in the natural system, but it varies based on how energetic the system is and how much it changes,” Trembanis said.
Going about the work
Designing a system framework that can be both trustworthy and ubiquitous for many situations is key. Having enough starting information also is important. According to Kien Nguyen, a third-year doctoral student in Peng’s lab, one problem with machine learning is that if there is insufficient training data, when you test a model on unfamiliar data, the model will struggle to perform as expected.
Nguyen gave the example of taking a model trained on seafloor mapping data from the Mid-Atlantic region of the United States and trying to apply the model to the ocean on another continent. While both areas of the ocean contain saltwater, sand and marine species, many other things could differ.
“When you want to apply the model to Asia or Europe, there are going to be inherent differences in the seafloor data, both subtle and explicit, and this makes the model confused," Nguyen said.
Trembanis recalled using a machine learning model that was robust in detecting scallops off the coast of Delaware, but “failed spectacularly” when used in a region of the ocean farther north. Understanding where these AI approaches fail, struggle or get things wrong are among the things the Navy wants to explore.
To overcome this issue, the researchers plan to gather a large-scale data set from existing surveys and then model the relationship between each cluster of data to provide more broadly representative data points from which the model can draw insights.
This is because, while machine learning is very good at detecting subtle patterns in large datasets, its weakness is that it doesn’t generalize well. For example, a person who has seen a cat will recognize another cat, even if it’s a different breed or color. But a researcher might need to feed a machine learning model 1,000 pictures of different cats for the model to recognize a cat.
The researchers will start by harnessing the existing data Trembanis has collected, along with publicly available data from the National Oceanic and Atmospheric Administration, the U.S. Geological Survey and other agencies and academic partners. Peng and Nguyen will develop new machine learning methods to optimize the model’s capabilities and then train and test the model on the datasets.
Through collaborative dialogue, Trembanis will assist Peng and Nguyen to understand when the model identifies things correctly and where it gets things wrong, based on his previous work. In this way, the researchers can improve the model’s ability to identify objects when deployed on data it has rarely or never seen, while ensuring the model’s decision-making process on unseen data is well understood.
Creating benchmark data
Getting started includes prepping the datasets to be AI-ingestible into Peng’s systems. It’s not just ones and zeros either. It could be time-series images of a specific place, like Redbird Reef, an artificial reef situated in the Atlantic Ocean off the coast of Delaware. It also could include sonar data or environmental data collected on conditions, such as wave speeds, water temperature or salinity, from a single site or across multiple sites or time periods.
“You can fire up your phone and type dog, boat or bow tie into a search engine, and it's going to search for and find all those things. Why? Because there are huge datasets of annotated images for that,” Trembanis said. “You don't have that same repository for things like subway car, mine, unexploded ordnance, pipeline, shipwreck, seafloor ripples, and we are working to develop just such a repository for seabed intelligence.”
Peng expressed excitement at the opportunity to improve the availability of this type of data.
“In the AI domain, there are a lot of people doing artificial intelligence, and they want to apply AI models for scientific applications, but many times suitable datasets don’t exist,” he said. “This project may fill this gap by generating some benchmark data for seafloor analytics. We want to improve the models, but also generate data for the machine learning and scientific communities.”
The researchers plan to link their AI-ready multi-site seabed database with other efforts in this area, such as the Ocean Biogeographic Information System and Seabed 2030, to improve data availability for a broad range of community stakeholders.
Beyond defense applications, the researchers hope their efforts can support decision-making on climate change, coastal resiliency or other efforts.
“You have commercial companies that are trying to track pipelines, thinking about where power cables will go or offshore wind farms, or figuring out where to find sand to put on our beaches,” said Trembanis. “All of this requires knowledge about the seafloor. Leveraging deep learning and AI and making it ubiquitous in its applications can serve many industries, audiences and agencies with the same methodology to help us go from complex data to actionable intelligence.”
Contact Us
Have a UDaily story idea?
Contact us at ocm@udel.edu
Members of the press
Contact us at 302-831-NEWS or visit the Media Relations website