
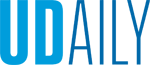

Data science leader
Photo by Evan Krape February 18, 2021
Cathy Wu, director of UD’s Data Science Institute, discusses the future of this fast-growing field
A tidal wave of data seems to flow from us and around us every day. The University of Delaware’s Cathy Wu is helping the world figure out how to handle, protect and make sense of it all.
Wu, Unidel Edward G. Jefferson Chair in Engineering and Computer Science, leads UD’s Data Science Institute, a hub for interdisciplinary research and collaboration, where faculty and students are exploring big data questions in business, health, energy, astronomy, policy, communications and many other fields.
She recently was named to the Association for Computer Machinery’s 2020 Class of Fellows for her many “contributions to bioinformatics, computational biology, knowledge mining and semantic data integration.” This is ACM’s highest honor and an achievement accorded to fewer than 1% of the Association’s 100,000 global membership.
A pioneer of international databases that are advancing discoveries in human health and other fields, Wu was recognized in 2019, for the seventh consecutive time, as a Highly Cited Researcher. She also serves on advisory boards at the National Institutes of Health, shaping data science’s future.
UDaily asked Wu for her thoughts about what’s happening now on the data science scene, and what’s coming.
Q: How do you define data science?
Wu: Data science essentially means extracting something of value from data. That’s why these big data companies, such as Amazon and Google, want to collect so much information on you. They can mine a lot of knowledge from all that data.
Q: How fast is data science growing as a career field?
Wu: LinkedIn named data scientist the number-one most promising job in America in 2019 and estimated that 11.5 million jobs in data science will be created by 2026. Glassdoor has also listed data scientist as one of the best jobs in America for the past several years. People want these jobs because they provide both a high salary and high job satisfaction.
Q: How is UD preparing the future data science workforce?
Wu: UD is doing a really wonderful job through its data science programs — we have a master’s program, and we’re also introducing a 4+1 program, where students pursuing a four-year degree stay on and complete their master’s course work in just one additional year. Even at the undergraduate level, there is now a minor in data science. We offer opportunities for students at any level.
Q: How far have we come in our capacity for data analysis?
Wu: Supercomputers were introduced in the 1960s. In the 1970s, the Cray-1 supercomputer had a peak performance of 250 megaflops (1 megaflops = 106 floating-point operations per second). Today, the fastest supercomputer in the U.S. — Summit at the U.S. Department of Energy’s Oak Ridge National Laboratory — is capable of 200 petaflops (1 petaflops = 1015 floating-point operations per second). Since June 2019, only petaflops systems have been able to make the world’s Top 500 list of supercomputers. But in 2021, an exascale supercomputer — the Frontier supercomputer — will debut at Oak Ridge National Laboratory, with a target computation performance at 1.5 exaflops. That’s 1018 or a quintillion flops. UD’s Sunita Chandrasekaran is leading one of only eight teams of scientists selected nationally to develop applications for this new machine. The exponential increase in memory, storage and computing power made possible by exascale systems will enable data intensive analytics and drive breakthroughs in scientific discovery and national security, from precision medicine, additive manufacturing, chemical and materials design, to energy production, earthquake risk assessment, discovery of fundamental forces of the universe and myriad others.
Q: How does our ability to secure data and systems affect the future of data science?
Wu: The security of data is absolutely essential. UD is actively looking into cybersecurity from both the engineering and the financial services side, which is a very important relationship, and the new Fintech building to be constructed at STAR Campus underscores that. While we are establishing this initiative at UD, the leaders in these areas are actively engaged with national and international organizations because of the coordination required across government and the private sector.
Q: With the rapid advance of technology, how do we keep up, and keep data useful? Are there security concerns to consider here?
Wu: Data science and artificial intelligence are considered high-priority development areas for our nation. The National Institutes of Health, for example, has a data science strategy based on being FAIR — Findable, Accessible, Interoperable (meaning useful across computer systems and software) and Reusable. The UniProt database, which my team and I are involved in, has been recognized as an early example of FAIRness. It means that a researcher funded by NIH may collect data for one purpose, but the data may be used in other ways the originator never really imagined. This applies to all sorts of data — geo data and sensor data, all the wearables. When these data sets are disseminated in a way that can be used for different purposes, that’s when data science becomes extremely powerful. One timely example of this is how our biomedical data is shared, and it raises important security concerns. Some data science infrastructure, such as Google/Verify and Microsoft cloud platforms, have been built on the principles of being open-source and standards-based, including standards set by the Global Alliance for Genomics and Health (GA4GH). GA4GH provides a data security toolkit with a framework for responsible sharing of genomic and health-related data. This is a great model for the data science community and for UD to adopt.
Q: How are artificial intelligence (AI) and machine learning in play at the Data Science Institute?
Wu: These methodologies actually have been in existence for decades and are re-emerging because of all the data. Artificial intelligence means giving computer systems the ability to do things typically requiring human intelligence, such as recognizing faces, translating languages and so on. Machine learning is a subset of AI, where computer algorithms and statistical models provide a training set of data that, with each correct answer, allows a computer system to become “smarter” at recognizing patterns, such as analyzing images of the brain and spotting a disease state earlier than humans can. Collectively, these tools can really drive science and technology forward. Many of our resident faculty and affiliated faculty at the Data Science Institute are working on fundamental algorithm development and applications of AI and machine learning.
I’ll mention just a few of them:
Explainable AI — Xi Peng is working on the robustness and transparency of AI systems. The goal is to produce “glass-box” (versus “black-box”) models, meaning models that humans understand why and how they work. In AI, this is called putting “humans-in-the-loop” to increase the trustworthiness of AI decisions.
Public Health Epidemics — Rahmat Beheshti is using AI to study social, economic, environmental and biological factors affecting major public health epidemics such as obesity and diabetes.
Brain Imaging — Austin Brockmeier is developing new machine learning approaches that more clearly identify patterns in the brain’s activity that relate to specific processes such as speaking and listening, moving and sensing, in both healthy individuals and in cases of chronic disease.
Urban Science — Gregory Dobler is applying data analysis techniques from astronomy, computer visualization and machine learning to images of urban skylines to study air quality, energy consumption, lighting technology, public health and sustainability.
Geospatial Data Science — Jing Gao is investigating large-scale interactions between humans and the environment, especially the relationship between land use, population and climate change. She integrates data and methods from spatial statistics, machine learning, big data mining, geo-visualization and remote sensing.
Understanding the Universe — Federico Bianco is using data science, AI, and big data across domains, from astrophysics to public policy. Trained as an astrophysicist, her work includes the study of stellar explosions, known as supernovae, and of pollution and the usage and distribution of energy in urban areas.
Q: What is your biggest concern about the future of data science?
Wu: The democratization of data science, the gap between the haves and the have nots — in the knowledge of how to analyze and interpret data — is getting bigger. How do we bridge that gap and also ensure we involve people who otherwise would be left out of the data and the decisions based on it? How do we prepare people for future changes in their jobs as data science evolves? We have to ensure data science really provides a positive impact to society, which requires attention to ethics, policy and education.
DSI resident faculty are tuned in to these issues and working to improve our ability to correlate and learn from data. Here’s a roundup of some of their activities:
Education and health care:
- Roghayeh (Leila) Barmaki is using machine learning, data science and virtual technologies to design advanced methods, software and tools for users to better interact with education and health care systems.
- Zachary K. Collier is advancing methods to better estimate the impact and outcomes of educational intervention. He is using machine learning techniques to improve the validity of educational research by reducing bias due to variables that can distort the relationship between educational interventions and student outcomes.
Learning from data:
- Cenchen Shen uses machine learning and statistics to find relationships within big data, such as between brain activity and personality, brain activity and certain diseases, social networks and personal activities, and more.
- Xiugang Wu investigates how to communicate and learn from data in our increasingly connected world. As a theorist, he is developing ways to extract information from large data sets distributed across various networks, for example, the internet of things or cloud computing applications that enable virtual home assistants, such as Siri.
Environmental understanding:
- Kyle Davis uses geographic and spatial data to understand how the global environment is changing, particularly our food systems and agricultural sustainability. With agriculture covering over 30% of the planet's land area, finding ways to produce more food while reducing agriculture’s environmental impact is key to a more sustainable future.
- Pinki Mondal examines the interaction between natural and human systems. Her current work focuses on investigating climate change impacts on forest, farmlands and wetlands and adaptation of vulnerable communities across the world using machine learning, satellite remote sensing and geographic information systems (GIS).
Q: What do you think the data science field will look like in 10 years?
Wu: Just to think five years from now, my head is spinning. I will say that one key conviction I have, and that my research group has — in whatever we do, we want to broaden and maximize the impact of our work. When we develop a new database or computational tool, we create a training package to make it easier for people to use it. That’s kind of a signature of what we do, to make sure whatever we develop becomes a resource for the community.
Contact Us
Have a UDaily story idea?
Contact us at ocm@udel.edu
Members of the press
Contact us at 302-831-NEWS or visit the Media Relations website