
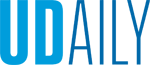

Shedding light on dark matter
Photo courtesy of Christopher Tunnell September 18, 2019
UD computer scientist co-leads $1 million project to develop methods to identify and localize dark-matter particles
Dark matter makes up an estimated 85 percent of the universe, according to scientists. But, despite years of study, scientists still do not know what dark matter is. One of the great challenges for physicists is to reconcile that quandary.
An interdisciplinary research team co-led by Hagit Shatkay, a professor in the Department of Computer and Information Sciences at the University of Delaware, has received a $1 million, two-year grant from the National Science Foundation (NSF) under the “Harnessing the Data Revolution” initiative, to develop computational methods to accelerate data-intensive discovery in astroparticle physics — an important step toward understanding dark matter. The project is planned as a case-study in domain-enhanced data science that will be applicable and extended to other data-intensive scientific fields such as meteorology and oceanography.
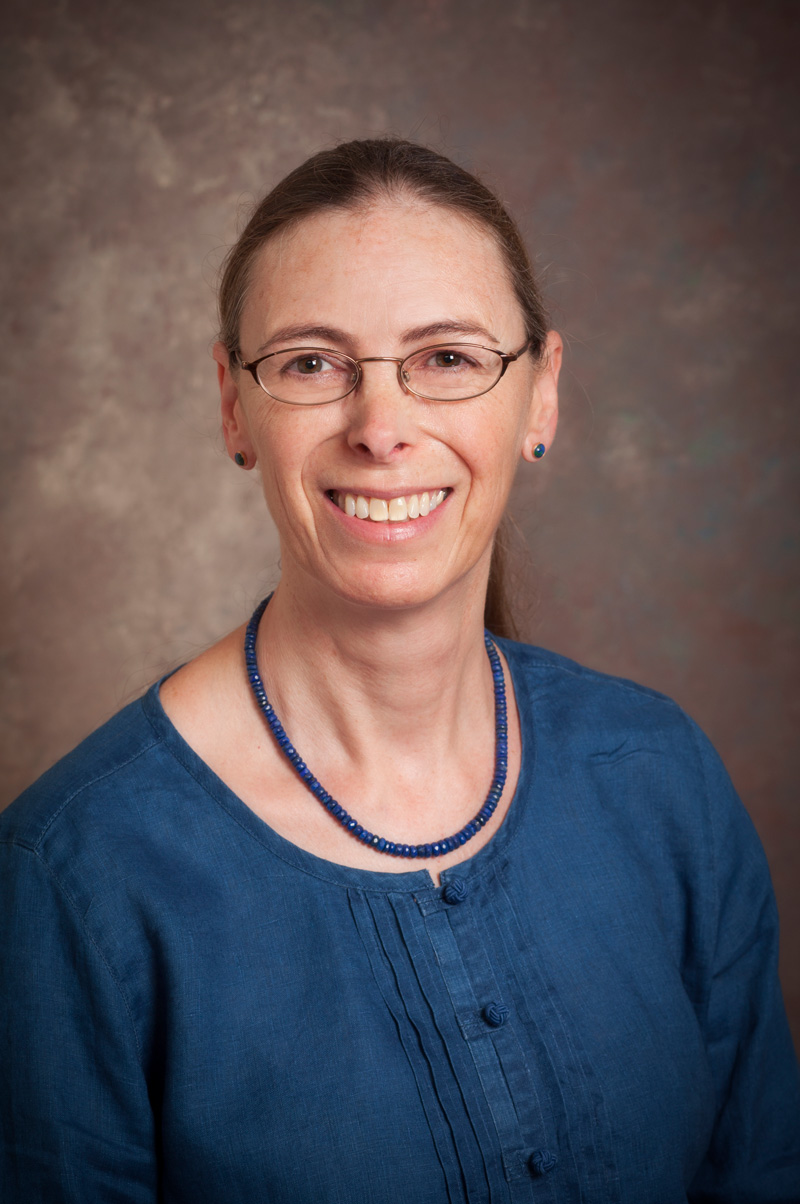
By developing methods for analyzing the data collected through noisy sensors at an underground experimental facility at the Gran Sasso National Laboratory in Italy, the newly formed team aims to help XENONnT and experiments like it detect, identify and localize dark-matter particles. The rich data sets generated by these sensors provide an ideal development ground for computational methods that could be useful well beyond particle physics. The research team anticipates that their methods will be broadly applicable to other scientific fields in which large quantities of noisy data is gathered from sensors in the hope of deciphering phenomena that we cannot directly observe or comprehend. As these sciences become increasingly data intensive, computational methods that incorporate domain knowledge will be required to make sense of the data.
Shatkay, a principal investigator, shares the grant with principle investigators Christopher Tunnell, an assistant professor of physics, astronomy and computer science at Rice University, and Waheed Bajwa, an associate professor of electrical and computer engineering and of statistics at Rutgers University. Tunnell will lead the project as he is a member of the XENON collaborative experiment, aiming to detect dark matter.
The collaboration between Shatkay, Tunnell and Bajwa resulted from discussions at an NSF IDEAS Lab in May 2019. At this week-long event, a select group of scientists from a wide range of disciplines and career stages put their heads together to push the boundaries of knowledge in data science. “This in itself has been an exciting discovery process through which we have formed an enthusiastic and diverse collaborative team,” Shatkay said.
The team formed after Shatkay spoke with Tunnell about his research on neutrinos, which are subatomic particles with no electrical charge and very low mass, that are abundant in the universe. Shatkay, an affiliated faculty member at UD’s Data Science Institute and in the biomedical engineering department, happened to be quite familiar with the topic — her sister was on the Sudbury Neutrino Observatory (SNO) team as a postdoctoral fellow.(SNO’s principal investigator was co-awarded the Nobel Prize in Physics in 2015, for demonstrating that neutrinos do have mass.)
Shatkay realized that her expertise in classical statistical machine learning, paired with Tunnell’s expertise in particle physics, could lead to innovative and unexplored ways of tackling unsolved problems in the field. Bajwa brings complementary expertise in inverse problem formulation, a method by which one begins with resulting observations and works backward, incorporating domain knowledge to calculate the causal conditions. The group is proposing the use of foundational probabilistic graphical models, machine learning and inverse problems to study dark matter, and they are ideally positioned to make advances in both data science and particle physics.
The team aims to inject domain knowledge from physics into machine learning methods, a type of artificial intelligence in which patterns are elicited by a computer through examination of vast amounts of data.
The data in this case is particle physics data collected by relatively sparse and noisy photo-sensors arrays. Gaps between the sensors form a non-contiguous coverage of the apparatus, and various sources of noise make the detection of the tiny particles – even in this highly advanced experiment – challenging. The use of probabilistic models can help account for the noisy and incomplete information, while directly incorporating the information and the knowledge that we do have.
“Typically, machine learning aims to train computers to perform tasks that are mundane or tiresome from available ground-truth data,” Shatkay said. “Often, the sought for ‘signal’ is known and well understood. For instance, we may have a lot of images showing mountains and images showing non-mountains and would like to have a computer learn to detect mountain images. Or have information about consumers that prefer one product over the other and attempt to identify consumers with similar preferences. In this project we tackle a very different machine-learning scenario: we want to identify phenomena that we do not directly know and cannot observe, based on very faint signal. In this case, we do not have ground-truth, the sensors we have are noisy, yet we are still trying to deduce what is out there – and where it is.”
In writing their proposal, the team noted that while dark matter may seem esoteric in everyday life, the research is highly important.
“Basically, given that dark matter comprises the majority of the Universe and we [living things] are in the minority — the question is not so much why we should care about dark matter but why should dark matter even care about us?” said Shatkay. On a more serious note, the research is highly applicable to other fundamental sciences, as evidenced by the letters of support received from prominent researchers in disciplines such as meteorology, climatology and oceanography.
The team plans to hold workshops to share their findings with and gather input from scientists in a wide range of data-rich disciplines. “When you think about what’s happening on the ocean floor, or in the atmosphere, what organisms, objects or movements are there, it’s the same situation,” said Shatkay. “You are trying to identify and localize those tiny items where you cannot access, cannot see, and all you have is a lot of data gathered by sparse and noisy sensors. One needs to incorporate every bit of available domain knowledge into the computational tools in order to make use of this data.”
Contact Us
Have a UDaily story idea?
Contact us at ocm@udel.edu
Members of the press
Contact us at 302-831-NEWS or visit the Media Relations website