
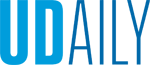

In pursuit of lifelong AI
Photos by Kathy F. Atkinson April 17, 2025
Keynote, panelists discuss adaptability, collaboration needed in the age of artificial intelligence
Eric Eaton, an associate professor of computer science at the University of Pennsylvania, hopes there will be a robot to help take care of him in the future when he is old. Mind you, he wants the robot to do more than learn to put the dishes away, and he doesn’t want to spend all his time training it. Rather, Eaton is after a robot that can learn and adapt to his needs, allowing him to age in place even as his requirements change over time.
This type of capability relies on what’s known as adaptive artificial intelligence, said Eaton during his keynote address at the University of Delaware Data Science Symposium on Monday, April 7. While it may seem far-flung, Eaton believes the current speed with which data science and artificial intelligence (AI) are evolving indicates it’s not really too much to ask.
Only a few decades ago, “Clippy” — a virtual office assistant whose avatar resembled a paperclip — was helping Microsoft users write letters or remember where they’d parked files on a computer. That early effort in artificial intelligence met with mixed approval in the 1990s.
Today, however, humans inherently rely on AI-enabled technology, such as voice-activated personal assistants like Google or Siri that can help users get directions, convert tablespoons to ounces, stream the latest musical hits and more. And while you are reading this, the large language models and generative AI powering ChatGPT, Gemini, CoPilot and other platforms are getting faster and smarter at any number of formerly human-only tasks.
That said, while AI is quite good at tasks it’s seen before, it still struggles with new things. According to Eaton, improving this poor performance through “persistent and adaptive intelligence” can enable advances with the potential to foster better financial prediction, enable more precise medical decision support and advance understanding of consumer preferences, to name just a few.
Getting there, he said, will require two-system models that excel in high-level tasks but also can generalize, multitask and adapt on the fly the way humans do.
Workforce training critical
The symposium’s panel discussion with experts from across the field identified collaboration between academia, government and industry as key to fully realizing the power of data science and AI in our lives. Workforce training, the panelists said, will be critical, both for students and for practitioners already in the field.
Students can best prepare themselves by becoming knowledgeable about the most current tools available, developing more than one skill and learning how to ask the right questions, the panelists said. In other words, be interdisciplinary and stay curious.
“This is an evolution of the workforce today … but the same thing happened with the steam engine and other big innovations,” said Harry Wang, UD professor of management information systems.
Specializing, it seems, is no longer enough. Those who excel, Wang continued, will be those who can do the coding, upgrade a product and know what the customer’s needs and tastes are today and successfully predict what they will be in the future.
Anjalie Field, an assistant professor of computer science at Johns Hopkins University, added that “it’s not just about how to solve a problem, but also about how students communicate their work to public audiences.” This includes how they engage with practitioners in other fields who might apply data science and AI tools to their own work.
Asked about the plausibility of AI-gone-rogue scenarios from movies like HER or Terminator, Eaton said he’s more concerned with what people do with AI systems, than what AI systems do themselves.
“One of my biggest concerns is a loss of privacy related to how the actual technology works,” Eaton said. “Ethical safeguards will be needed.”
It’s a valid worry and something that researchers and practitioners in the field are already thinking about.
One health care clinician in the audience wondered how AI could be trusted to positively impact health care to a population or to a specific patient when most AI and machine learning currently relies on retrospective analysis of data. How should users evaluate answers that AI models suggest?
The panel responses were thoughtful:
Yes, bridging AI and clinical expertise is needed, and will continue to be needed as new methods, data and discoveries in AI continue to evolve.
Yes, AI models will continually require evaluation to ensure they perform as designed/desired.
Yes, proper oversight will be needed.
Moreover, users should evaluate the answers AI models suggest the same way they evaluate responses from humans in their everyday personal or professional interactions … with critical thinking and active validation.
In terms of clinical applications, Field suggested incorporating AI in settings that minimize the ability of a model to be incorrect might be a good place to start.
“Using AI to listen to a patient interaction and write a summary, then having the doctor review, edit and correct the summary is a way to save time with reduced room for error because of human involvement,” Field said.
Charting the future of AI
As the world works to adapt and apply the advances in AI to their personal and professional lives, one thing remains certain, people remain at the center, at least for now.
Consider the many student studies underway at UD using data science and AI to explore solutions for societal problems ranging from colorectal cancer to inherited retinal disorders, food protection, water quality, plastics waste, menopause, pharmaceuticals, cancer and other human diseases.
There is power in these studies — and in the researchers conducting them.
Tools like AI can complement this human ingenuity with more and different types of models working together, according to Eaton.
“How the models work together will be important and this will allow us to choose and solve different scale problems,” he said.
But that doesn’t mean AI tools will replace humans. Leveraging AI and data science is just part of the equation.
And it turns out, human connections can be pretty powerful.

UD doctoral candidate Manju Anandakrishnan showcased her work using data science and AI to identify the interconnectedness of UD researchers on campus, as part of the Data Science Institute’s student networking competition.
Her project analyzed the collaboration network of UD faculty using data about their co-authored publications from 2016-2024. The data, which included 549 faculty from eight colleges and 53 departments, revealed that of the 2,300 publications identified from the eight-year period, 1,085 papers were found to be co-authored by at least two UD faculty.
Mapped on a graph known as a social network diagram, it was possible to see all the connections an individual faculty member had, based on co-authorship, and — diving deeper — the second-level connections made possible through their peers’ connections. This brief illustration showcased how interconnected one person’s world can become. Viewed at a larger scale, say all faculty at an institution or all the people in a given community, the potential connections become endless.
Ultimately, it's this collaborative brain power — combined with technology like data science and AI — that will usher in solutions to today’s grand challenges facing health care, finances, the environment and more.
“Machines are better than humans for many things, such as chess, golf, etc. … but you still need humans,” Wang said.
Contact Us
Have a UDaily story idea?
Contact us at ocm@udel.edu
Members of the press
Contact us at 302-831-NEWS or visit the Media Relations website