
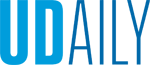
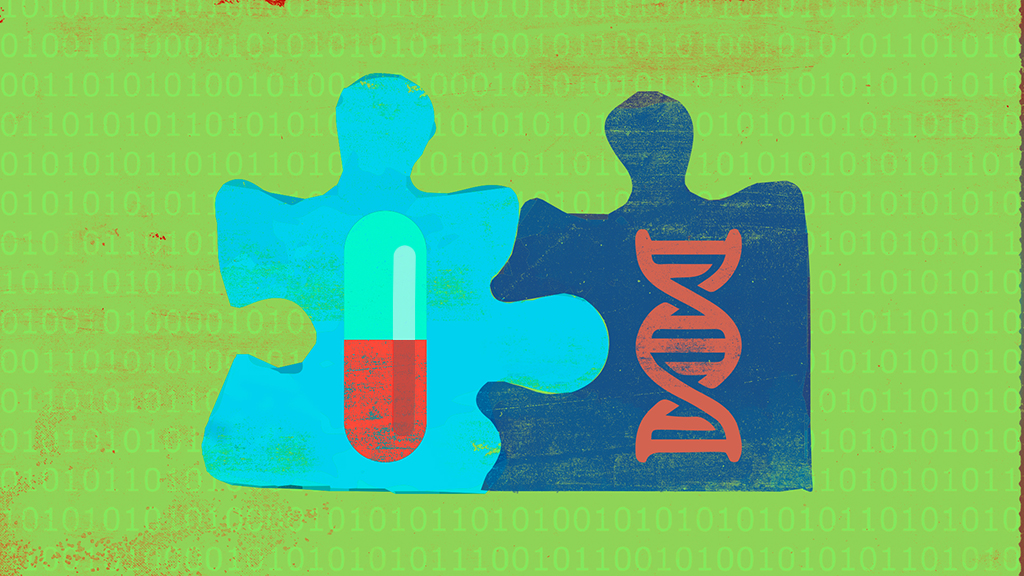
Matchmaking for cancer care
Illustration by Jeffrey Chase February 01, 2018
New system matches genetic anomalies with precision medicine treatments
A team of computer scientists from the University of Delaware and Georgetown University has developed a new system to rapidly determine which cancer drugs are likely to work best given a patient’s genetic markers. The first publicly available system of its kind, their database, eGARD (extracting Genomic Anomalies association with Response to Drugs), is described in PLOS One.
When your genes work correctly, they function like miniature factory plant managers, directing the production of life-sustaining proteins. But sometimes, a gene goes rogue and manufactures a cancerous tumor instead. When cancer experts identify these faulty genes, they can devise treatment plans based on past evidence.
However, until now, the data linking genetic factors and treatment results has been spread among hundreds of academic journals. It would take days for doctors, doing nothing else, to find and read all these reports. Now, they may be able to spend that time delivering optimized treatments instead.
The promise of eGARD
eGARD is a text mining system that analyzes words and phrases in medical literature to find relationships between genomic anomalies and drug responses.
“Clinicians have no time to read all of the reports and literature for each tumor,” said Peter McGarvey, a study author and associate professor of biochemistry at Georgetown University. “eGARD is a way to help surface the important ones for clinicians, medical geneticists or maybe companies that already are doing this in other ways.”
The research team applied eGARD on roughly 36,000 article abstracts, retrieving 50 genes and 42 cancer drugs, including cell cycle inhibitors, kinase inhibitors and antibody treatments.
The research team first trained the system to identify indications of genetic anomalies, with very scientific names such as “over-expression of ERCC1” or “C677T and A1298C polymorphisms of MTHFR gene.” Then, they trained it to look for text suggesting treatment outcomes, such as “significantly poorer response” or “survival rate.” Next, they sought words and phrases connecting a genetic anomaly and outcome, such as “correlate,” “associate” or “sensitize.”
By extracting and processing key pieces of text, eGARD can match genetic signatures with outcomes with 95 percent precision.
“We hope this could make a difference for oncologists and cancer patients alike,” said study author Vijay Shanker, a professor of computer and information sciences at UD.
UD researchers developed the code and data processing for eGARD, and clinically focused researchers at Georgetown provided use cases, terminology, curated datasets and insight on what information was most important to clinicians working in precision medicine. Both groups tested and refined the system.
The team will make a public interface for eGARD. It may also be incorporated into other software eventually.
The authors of the new paper include A.S.M. Ashique Mahmood, a doctoral student in computer and information sciences at UD; Shruti Rao, a research associate at the Innovation Center for Biomedical Informatics at Georgetown University; McGarvey; Cathy Wu, Unidel Edward G. Jefferson Chair in Engineering and Computer Science and director of the Center for Bioinformatics and Computational Biology at UD; Subha Madhavan, director of Biomedical Informatics at Georgetown University; and Shanker.
This project was funded by MACE2K as part of the National Institutes of Health BD2K (Big Data to Knowledge) initiative.
Some students involved with this project also had a chance to prove how well their system works in November at TREC 2017, the Text REtrieval Conference, held in Gaithersburg, Maryland.
A team led by Mahmood and Gang Li, also a graduate student in computer and information sciences, took some top honors in the precision medicine track.
Participants were given information about a patient’s disease, relevant genetic variants and other key factors. Then they were asked to retrieve clinical trial information and abstracts of biomedical articles relevant to the patient and judged on three measures of their ability to do each. In a competition with 32 teams, the UD team ranked first on all three measures related to clinical trials. For abstracts, the UD team earned rankings of first, fourth and fifth.
iTextMine for knowledge integration
eGARD is not the first or last system from this group of big data experts. A suite of text mining tools has been developed by students and research scientists over the years through the long-standing collaborations between Shanker and Wu. Funded by another NIH grant, the UD research team has developed the iTextMine (Integrated Text Mining System for Large-Scale Knowledge Extraction from Literature), which uses an automated workflow to run multiple text-mining tools on the entire PubMed with millions of citations for biomedical literature.
“By analyzing scientific texts with multiple text mining tools, researchers may further gain knowledge on gene-drug-disease relationships and better understand the underlying molecular mechanisms,” said Wu.
This tool allows users to browse the text evidence for multiple biomarkers and view integrated results through a network visualization. The iTextMine will be presented by Jia Ren, a student in the Bioinformatics and Systems Biology Ph.D. program, at the International Biocuration Conference in China this April.
Contact Us
Have a UDaily story idea?
Contact us at ocm@udel.edu
Members of the press
Contact us at 302-831-NEWS or visit the Media Relations website