
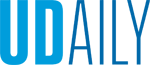
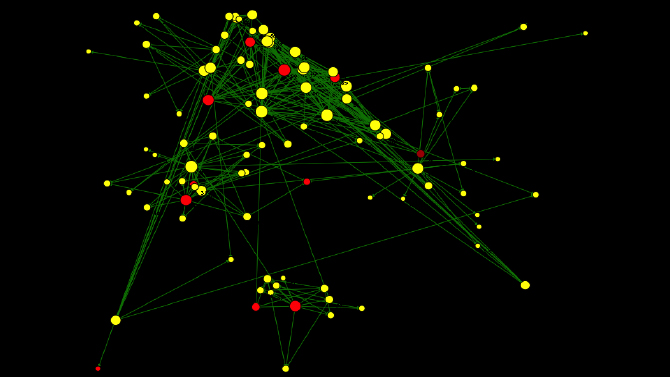
Predicting the impact of social networks
March 20, 2017
UD’s Xiao Fang uses analytics to understand, predict social media interactions
If you’ve ever bought an item that a celebrity recommended on Twitter or been persuaded by a discussion with a Facebook friend, you know that social media can impact your behavior and opinions. But you might be surprised to know that there’s a science behind social media interactions.
The University of Delaware’s Xiao Fang, associate professor of MIS, utilizes advanced data mining and machine learning methods to better understand these social media influencers and adopters.
“My research on social network analytics focuses on a unique phenomenon in social networks – the diffusion of adoption behavior (e.g., adoption of a product, service or opinion) from one social entity to another,” Fang said.
Fang’s work studies questions like:
• How do you predict adoption probabilities in social networks?
• How do you predict which social network users will be top persuaders?
• How do you make link recommendations within social networks?
“Solutions to these problems have direct implications in many critical real-world applications, from business (e.g., viral marketing) to politics (e.g., diffusion of political messages on Twitter),” Fang explained.
But studying social media’s impact on behaviors in the real world can present unique challenges, he said.
For example: While social influence does have an impact on decisions, Fang said, “There are also hidden factors that are key to adoption decisions.”
“Someone may see a billboard advertisement of the product when driving home, get interested and eventually purchase (i.e., adopt) the product,” he said. “Such factors are not recorded and hence hidden.
“The principal challenge for this problem thus is how to predict adoption probabilities in the presence of hidden factors that are generally unobserved.”
To address this challenge, Fang’s team developed advanced learning models using a sophisticated statistical inference method known as Bayesian inference. Their experiments with real-world data lead to two interesting observations:
1. Existing methods that exclusively use social influence to predict adoption probabilities seem ineffective.
2. Hidden factors appear to play a significant role in adoption probability predictions.
And Fang’s work in this area has received notable attention and acclaim.
During his first academic year at UD’s Lerner College of Business and Economics, Fang published three articles in elite management science academic journals: MIS Quarterly and Management Science. His research has been widely featured in the media as well, including MIT Technology Review and MIT Sloan Management Review.
Fang also received the 2016 Institute for Operations Research and the Management Sciences (INFORMS) Design Science Award in recognition of “excellence in research efforts centered on the design and realization of innovative information technology artifacts.” INFORMS is the largest society in the world for professionals in the field of operations research, management science and analytics.
On receiving the INFORMS Award, Fang said, “I feel the award is the right recognition of Lerner and the Institute for Financial Services Analytics’ strength in data science and business analytics, and my research endeavors in this area for almost 20 years.”
Contact Us
Have a UDaily story idea?
Contact us at ocm@udel.edu
Members of the press
Contact us at 302-831-NEWS or visit the Media Relations website